Author: Narasimha Kumar, CPO, Datafoundry
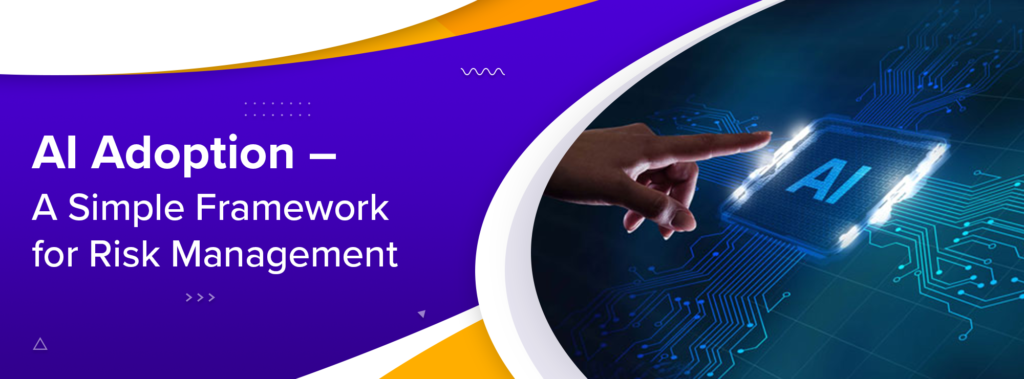
With Artificial Intelligence (AI) in general, and Generative AI in particular becoming the next big thing in technology, we see industries across sectors clamouring for the infusion of AI into their business processes for both insights and process automation.
However, in many regulated industries, there is a healthy skepticism regarding the real value of AI vis-à-vis the costs and risks associated with the implementation. Companies are cautiously optimistic – some PoCs are being approved, while the general tendency is to wait for the technology to mature so that business objectives from AI have a more than reasonable chance of success. At the same time, the fear of missing out (FOMO) on technology innovation and disruption, ceding an advantage to nimbler, risk-taking competitors, is driving company boards to ask the executive leadership the following question: What is our comprehensive AI plan?
In this context, it is important to assess the risks that could impact the success or failure of an AI transformation program and the mitigations that could improve the chances of success. This blog is a simple framework for navigating the process of AI adoption at enterprises of any scale and in any sector.
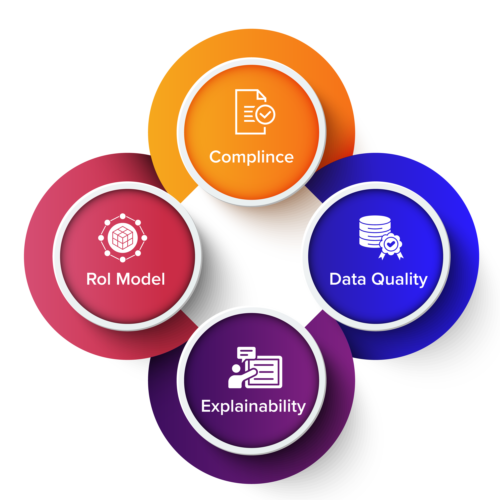
Firstly, any consultant would tell you the importance of ‘Data Quality.’ Even before the advent of AI in enterprises, the ‘data quality issue’ had been plaguing companies for years regarding data mining and business intelligence solutions.
Without getting into the reasons for data quality issues, low-quality data impacts data-driven decisions, with or without AI. Missing values or gaps in data, duplicate entries, outliers, data formatting errors, and variance in metadata usage are common problems due to historical reasons for information/software technology evolution and implementation.
So, as part of an AI Transformation initiative, having a clear plan for Data Quality Assurance is critical. Automation of data standardization through custom SQL rules or Machine Learning (ML) algorithms will significantly increase the success of AI/ML models in meeting feature objectives. The 4Vs of data quality - Veracity, Variety, Velocity, and Volume – must be assessed and ensured as a continuous program, with sufficient resources allocated. Data quality monitoring tools should be selected based on the organization’s needs, as they could range from simple data testing to comprehensive data observability platforms.
The second element of the framework relates to Compliance. Investment in AI transformation could be at risk if one fails to ensure compliance with industry standards/best practices, regulatory requirements, and guidance. Information security, data integrity, and privacy considerations need to be baked into the design of AI algorithms at the PoC stage itself instead of assuming that these will be taken care of when the time comes for production implementation and scaling. It makes business sense to expect compliance as part of the innovation process and not as a pre-production checklist. This approach will help align stakeholder expectations and appropriate risk mitigation or acceptance ownership. Building stakeholder trust in AI algorithms is key to success.
Any AI program should have a quality strategy or plan to enable effective and efficient compliance. This plan can evolve through the program’s life cycle but must be executed fully as part of going live—even if it is a PoC or an MVP.
The third element of the framework relates to the actual working of the AI-driven solution – in other words, the Accuracy and Explainability of the algorithms used. A thumb rule used in general, where AI algorithms replace human effort, is to assess the accuracy (F1 score) of the model compared to a manual process. Where it is a repetitive task of processing large data sets, the ML models outperform humans and eliminate manual processing errors. However, in use cases where the models establish relationships among data entities and perform analyses based on the relationship extraction, it is critical to have a human-in-the-loop to validate the AI predictions. The ML engineers and data scientists should also establish the explainability or interpretability of the models through a combination of tools and documentation. This might seem like an overhead, but if critical decisions are to be made based on AI recommendations, Explainable AI is non-negotiable.
The final element of this simple framework relates to the Cost-Benefit analysis or RoI model of the proposed AI solutions. Many consultants would say this is, in fact, the very first element of any business case approval. That could be the case. In reality, all these elements must be considered in a parallel process, and the way ahead should be mapped out as part of an AI Transformation program. Choosing the right automation technique is important. If I have to travel from my home to the airport in my city, I would either drive or take local transportation – I will not (unless I am a VVIP) usually take a jet plane. Using expensive computing, storage, and human resources to solve a problem that needs simple rule-based automation makes it a case of ‘AI for AI’s sake.’ The cost assessment should consider all aspects of the program from inception till steady state and value realization.
Like all technology initiatives, an AI adoption program will also have residual risks even after the best risk management approaches are in place. One should avoid long weeks of planning and analysis meetings by having the right SMEs collaborate and define the strategy for the program. There will be a tendency to create new functions and teams, making some people feel left out despite being the right people to contribute. This situation is prevalent in larger organizations. The solution is simple – effective communication across the organization and using collaboration tools to access the organization’s collective wisdom efficiently. Though this approach is not specific to an AI Program, it applies to any transformation initiative.
Datafoundry is a crucial partner in navigating AI adoption and offers robust solutions to enhance data quality, ensure compliance, maintain accuracy, and optimize cost-effectiveness, ultimately driving successful AI transformation. For further insights and to share experiences or challenges faced in AI adoption, please contact Narasimha Kumar, Chief Product Officer at Datafoundry, at narasimha.k@datafoundry.ai.