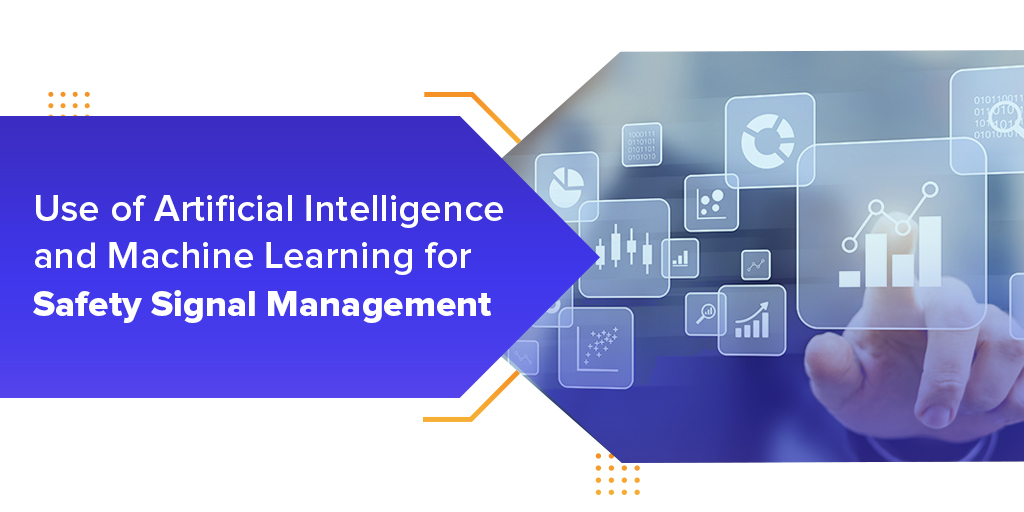
Detecting and understanding safety issues associated with drug can be achieved through early surveillance and appropriate risk minimization measures. It is a lifelong activity for the drug, designed to ensure early detection of safety signals, identify and mitigate the risks, and minimize the likelihood of possible safety issues.
PV signal detection has been traditionally performed using manual and semi-automated means based on statistical analyses and/or expert judgement. Although useful for generating signals, these methods often fail to identify signals that require further investigation as they are limited to quantitative data and conventional statistical tools, which cannot capture all types of patterns in vast amounts of complex heterogeneous data such as that generated from E2B(R3) ICSRs, literature, social media, data from clinical trials and electronic health records (EHRs).
Artificial Intelligence (AI) and Machine Learning is increasingly used across the pharmaceutical industry for a wide range of applications where computers can provide more accurate results than humans, e.g., drug discovery, medical imaging, and pharmacovigilance (PV) signal detection. For safety signal management (SSM), AI techniques can be applied to improve the early detection from multiple sources for a potentially serious threat, as well as improve PV performance metrics (e.g., timeliness and completeness).
Improving identification and classification:
AI-based approaches are well–suited to address the challenges of proper identification and classification of the severity levels of safety alerts. Because the large amount of historical and real-world data on safety issues and the need for standardised decision tree processes can be delivered effectively via Machine Learning algorithms. By using advanced analytic techniques, huge data can be grouped better, and associations can be identified near to perfection.
Predictive signal detection:
AI can assist the signal evaluators to make more informed observations with data-driven algorithms. For example, by using statistical methods like BCPNN, Multivariable logistic regression analysis predicting for selection and prioritization of potential signals. This suggests that AI can increase timeliness and completeness by identifying patterns in the data that would not have been detected by traditional methods.
Safety experts are likely to get lesser trial data (due to expedited regulatory approvals) and more real-world data (due to increased reporting requirements) than in the past, so predictive signal detection (using AI/ML technology) is a valuable tool.
Better evaluation of risk-to-benefit ratio:
For better risk-benefit profiles and to reduce the time required to create such profiles, risk evaluators are increasing the number and types of datasets with which they conduct signal detection. As a result, they need the help of ML algorithms to understand the differences between the signal scores across multiple datasets, including the method for summing them. The aggregated case data provide a more holistic picture of signals than any single case, and this aggregated data allows for signal discovery-looking for patterns that may indicate previously unknown risks.
Better analytics:
The use of Artificial Intelligence (AI) and Machine Learning is increasingly used for better analytics in safety signal management. Different types of data streams have been used in recent research to gain a more complete picture of specific safety issues that appear in the same or similar databases. Analytics capabilities are expanding to take advantage of these new data streams as the number of inputs increases. Faster and better analytics makes it easier for the reviewers to detect cases of interest by prioritizing those flagged as higher risk.
The Road ahead
Using Artificial Intelligence (AI) and Machine Learning (ML) for safety signal management (SSM) is a promising approach that has already started to be implemented in various pharmaceutical companies. AI and ML powered safety systems appear to be suitable for detecting signals and proper classification. They simplify the “heavy manual component” often associated with traditional signal management systems and bring speed, consistency, and efficiency as well as predictive capabilities. Automating the manual and time-consuming process of signal management can free time for experts to focus on more complex activities.
Datafoundry’s Signal Management Solution
A new age solution from Datafoundry brings in the power of AI and automation for Signal Management- DF mSignal AI – a safety signal management solution for pre and post-marketing surveillance. It uses advanced algorithms to automatically collect, monitor and analyze safety data, for superior and efficient signal detection and management. It is an end-to-end Signal Management solution that can be plugged into any standard safety database system. DF mSignals AI adheres to industry best practices and regulations with a user-friendly interface for easy navigation and drill-down analytics.