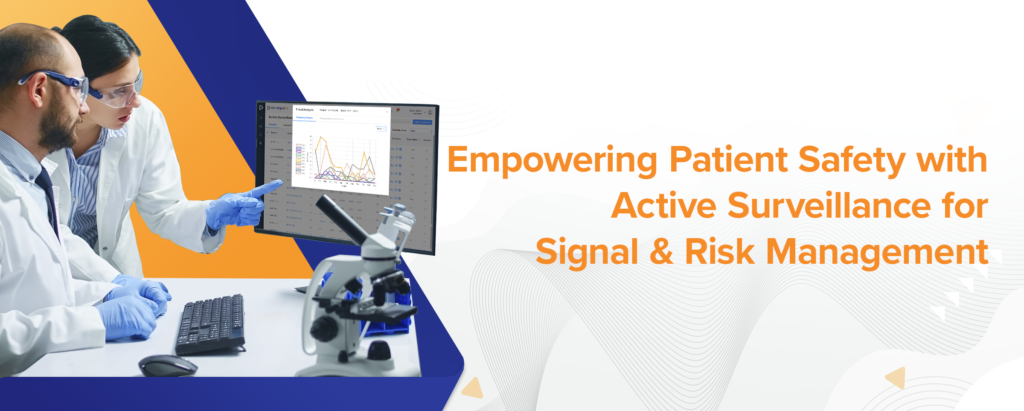
Patient safety is of the utmost significance in the healthcare industry across the world. Every person seeking medical care is entitled to the greatest degree of safety and quality in their treatment. The primary goals of healthcare providers, regulatory agencies, and other stakeholders involved in healthcare delivery are to prevent adverse events, minimise risks, and ensure optimal patient outcomes.
One notable initiative in this regard is the FDA Sentinel Initiative. Developed by the U.S. Food and Drug Administration (FDA), the Sentinel Initiative is a groundbreaking system that uses real-world data to monitor the safety of medical products throughout their lifecycle. By leveraging electronic health records, insurance claims databases, and other healthcare data sources, the Sentinel enables ongoing monitoring of product safety, efficacy, and potential risks.
Active surveillance is essential for safety vigilance because it allows for the rapid identification and handling of safety signals. Active surveillance entails the systematic and continual monitoring of data from a variety of sources, including clinical trials, adverse event reports, post-marketing surveillance, and real-world data.
This article aims to explore the significance of active surveillance in signal management for safeguarding patient safety. It will delve into the principles, strategies, and benefits of active surveillance, highlighting its role in preventing adverse events and improving healthcare outcomes. The article will also discuss the methods and technologies employed in active surveillance, along with the challenges and future directions in this field.
Understanding Active Surveillance
Active surveillance is a proactive strategy to monitor safety signals throughout the lifespan of a product. It goes beyond passive surveillance, which is based on the spontaneous reporting of adverse events, by actively obtaining and analysing safety data from various sources. The active surveillance approach detects and evaluates any safety risks by utilising innovative technology, data analytics, and dedicated professionals and has the potential to revolutionize patient safety.
Benefits of Active Surveillance
Active surveillance offers several significant benefits in enhancing patient safety:
- 1. Early detection of safety signals: Active monitoring allows for the early detection of safety signals, even before they are reported through the spontaneous reporting method. This early discovery enables rapid investigation and management, therefore reducing adverse outcomes and minimising patient harm.
- 2. Improved risk management: Healthcare providers and regulatory bodies may identify the risks associated with medical procedures and build effective risk management plans by regularly monitoring safety data. These tactics may involve changing product labelling, establishing risk mitigation measures, and, in extreme cases, pulling items from the market.
- 3. Enhanced post-marketing surveillance: Active surveillance supplements standard post-marketing surveillance by offering real-time data analysis and continuous monitoring. This method guarantees that safety issues are addressed immediately and that necessary steps are taken to protect patient well-being.
- 4. Accurate product effectiveness assessment: Active surveillance aids in assessing the efficacy of medical therapies by studying real-world data. This data may be used to make evidence-based decisions and improve patient care.
Various Considerations in Active Surveillance
The following are the various considerations used in active safety signal surveillance:
- 1. Data Collection and Integration: Active surveillance relies on extensive information obtaining from a variety of sources, including electronic health records, claims databases, social media, clinical trials, and the adverse event reporting procedure. The combination of different data sources gives a more complete picture of patient safety, allowing for a more accurate assessment of possible risks and safety signals. Advanced technologies are required for harmonizing the huge volumes of data from disparate sources.
- 2. Advanced Analytics and Machine Learning: Active surveillance relies heavily on advanced analytics techniques such as data mining and machine learning algorithms that enable risk flagging. These technologies enable pattern detection, trend identification, and safety signal prediction. Advanced analytics improves the efficiency and accuracy of signal identification by analysing massive datasets, enabling proactive risk management.
- 3. Collaboration and Information Sharing: Collaboration among multiple stakeholders, such as healthcare providers, regulatory agencies, and pharmaceutical corporations, is essential to active surveillance. Effective information exchange and teamwork enable a thorough comprehension of safety signals and allow for quick response. Collaboration allows for the exchange of knowledge, experience, and resources required for effective active surveillance.
- 4. Ethical Considerations and Privacy Protection: Active surveillance must follow stringent ethical rules and place patient privacy first. Patient confidentiality, informed permission, and the use of anonymised data are all critical. Ethical considerations play a vital role in fostering trust among patients, healthcare professionals, and other stakeholders, thereby enhancing the success and acceptability of active surveillance projects.
- 5. Continuous Improvement and Learning: Continuous improvement and learning are essential for the success of the active surveillance approach in a changing healthcare setting. To effectively adapt and stay relevant, the active surveillance strategy must undergo frequent reviews and monitoring to incorporate the latest learnings and insights.
By regularly evaluating the active surveillance strategy, healthcare professionals can identify areas for improvement, address emerging challenges, and capitalize on new opportunities. This proactive approach ensures that the strategy remains aligned with the evolving healthcare landscape and incorporates the most up-to-date information and evidence.
It is critical to evaluate techniques, data quality, and signal detection algorithms on a regular basis. Active surveillance stays efficient and precise in identifying safety signs by learning from prior events and using developments in technology and analytics.
Key Challenges and Future Direction
Below are some key challenges and future directions in active surveillance in signal management:
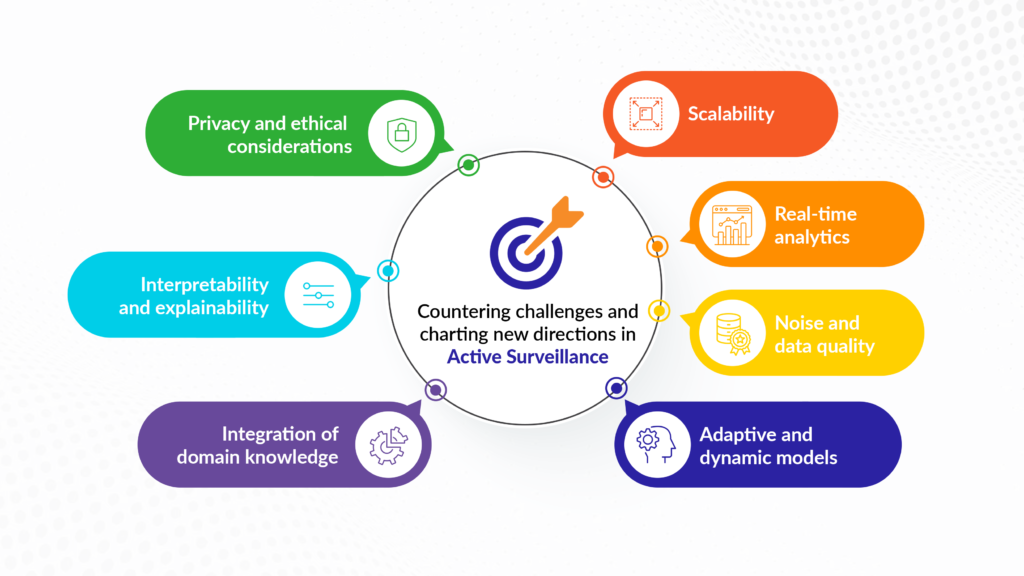
- 1. Scalability: Scalability becomes a challenge when the volume, velocity, and diversity of data continue to expand rapidly. The active surveillance method must be capable of handling and operating large-scale data streams in real time. To address the scalability difficulty, future prospects include the development of distributed and parallel computing systems, stream processing frameworks, and optimised algorithms.
- 2. Real-time analytics: Real-time analytics poses challenges in active surveillance due to the complexities of managing and analyzing large volumes of healthcare data in real-time. The sheer data volume, encompassing patient health records, diagnostic results, and sensor data, requires robust infrastructure and advanced analytics capabilities. Additionally, integrating data from multiple sources and ensuring interoperability can be complex, affecting the timeliness and accuracy of real-time analytics. The active surveillance method should be able to do real-time analytics in order identify anomalies and trends as they occur. The next trend might include the development of stream processing methods, machine learning algorithms, and hardware acceleration to enable real-time analytics on high-speed data streams.
- 3. Noise and data quality: The signal identification process frequently encounters noisy and low-quality data. In anomaly detection and pattern recognition, this can result in false positives or false negatives. Using preprocessing techniques, noise reduction algorithms, and outlier identification approaches to address data quality difficulties is a critical task. The use of data cleansing and data fusion techniques has the potential to increase the accuracy and reliability of the active surveillance process.
- 4. Adaptive and dynamic models: Changes in statistical features and underlying patterns occur in signals and data streams throughout time. To be effective, the active surveillance method must adapt to and learn from these changes. Future directions include the creation of adaptable models, online learning algorithms, and ensemble techniques that may dynamically modify their behaviour in response to changing data features.
- 5. Privacy and ethical considerations: The acquisition and analysis of sensitive data is frequently used in the active surveillance process, posing privacy issues. A significant difficulty is striking a balance between the requirement for monitoring and individual privacy rights. The development of privacy-preserving techniques such as safe multiparty processing, differential privacy, and federated learning can allow for effective signal management while adhering to privacy constraints.
- 6. Interpretability and explainability: As active surveillance processes get more complicated and rely on powerful machine learning techniques, interpretability and explainability become increasingly important for establishing trust and understanding their judgements. Explainable AI approaches may give explicit and transparent explanations of the logic behind surveillance results, allowing stakeholders to evaluate and confirm the results.
- 7. Integration of domain knowledge: Active surveillance processes can be improved by incorporating subject knowledge and human skills. The creation of hybrid processes that combine automated signal analysis with human input or override based on subjective evidence can increase detection accuracy and decision-making.
The Role of Healthcare Providers, Regulatory Agencies and Pharmaceutical Companies in Active Surveillance
Active surveillance strategies rely heavily on healthcare providers. Their active engagement includes reporting adverse events, exchanging real-world patient data, and giving clinical knowledge. Active surveillance by healthcare practitioners contributes to a more accurate evaluation of safety signals, thereby improving patient safety.
Whereas the regulatory agencies are critical in ensuring the safety of healthcare goods and procedures. Active surveillance allows regulatory bodies to continually monitor safety data, coordinate with other stakeholders, and respond to emergent safety problems in real time. The participation of regulatory bodies, as well as the active monitoring process, enables effective risk management and patient safety.
Active surveillance is also important to pharmaceutical businesses since it helps assure the safety and effectiveness of their medicines throughout their lives. Active surveillance enables them to address possible concerns proactively, update product labelling, and execute risk management methods. Working with regulatory bodies and healthcare providers creates a collaborative effort to improve patient safety.
Active surveillance is a valuable tool for safeguarding lives by detecting signals and managing risks in real time. Active surveillance improves patient safety and reduces the chance of adverse events by continually monitoring safety data, utilising advanced technologies, and encouraging teamwork. Active surveillance allows for early intervention, ensuring that appropriate steps are taken to safeguard patients’ lives and enhance healthcare outcomes, while maintaining a patient-centric approach at all levels.
As healthcare evolves, investing in signal management solutions and developing a culture of safety vigilance becomes increasingly important, emphasising the commitment to providing high-quality treatment and prioritising patient well-being. DF mSignal AI is a reliable signal management solution for pharmaceuticals, cosmetics, vaccines, nutraceuticals, and medical devices that provides advanced, accurate, and complete signal identification and risk management coverage.
The solution offers a robust suite of analytics and insights that empower configurable active and passive surveillance of signals related to drug-event combinations. It provides automated statistical scores by analyzing safety data from various sources, including regulatory agency portals and company safety databases. It enables thorough monitoring and detection of potential safety issues, enhancing the overall surveillance process. By leveraging advanced technology, it facilitates comprehensive signal analysis, offering valuable information and insights for improved pharmacovigilance and patient safety.